Multi-stained Pathology Image Analysis
model pretraining, cross-strain image generation, lymphoma classification
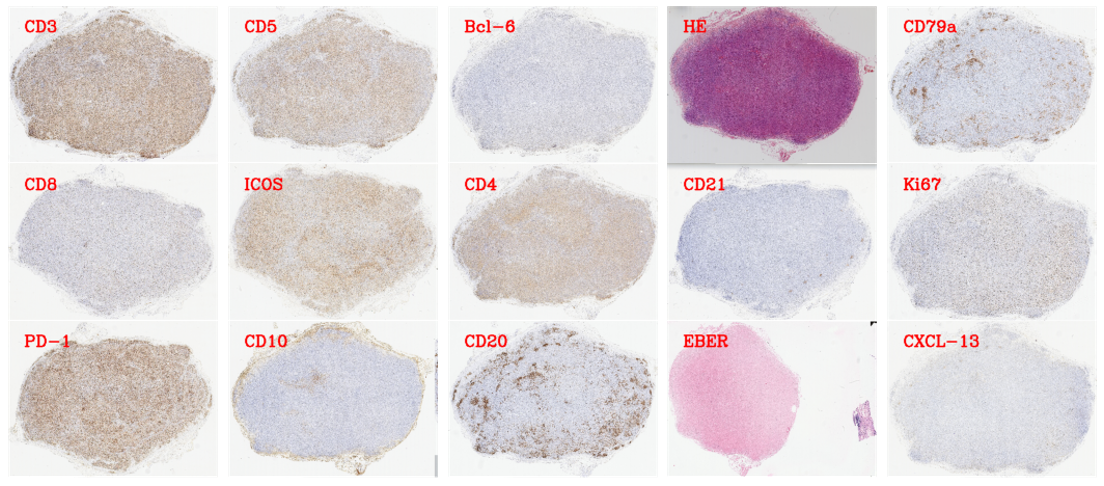
Hematoxylin and Eosin (H&E) stained images are the most common modality of pathological images, while accurate tumor subtyping is reliant on the information provided by various Immunohistochemistry (IHC) images.
We initially attempted qualitative and quantitative analyses on the PD-L1. The focus on the project of lymphoma diagnosis with both H&E image and tens of IHC markers, simulating the entire workflow of pathological diagnosis.
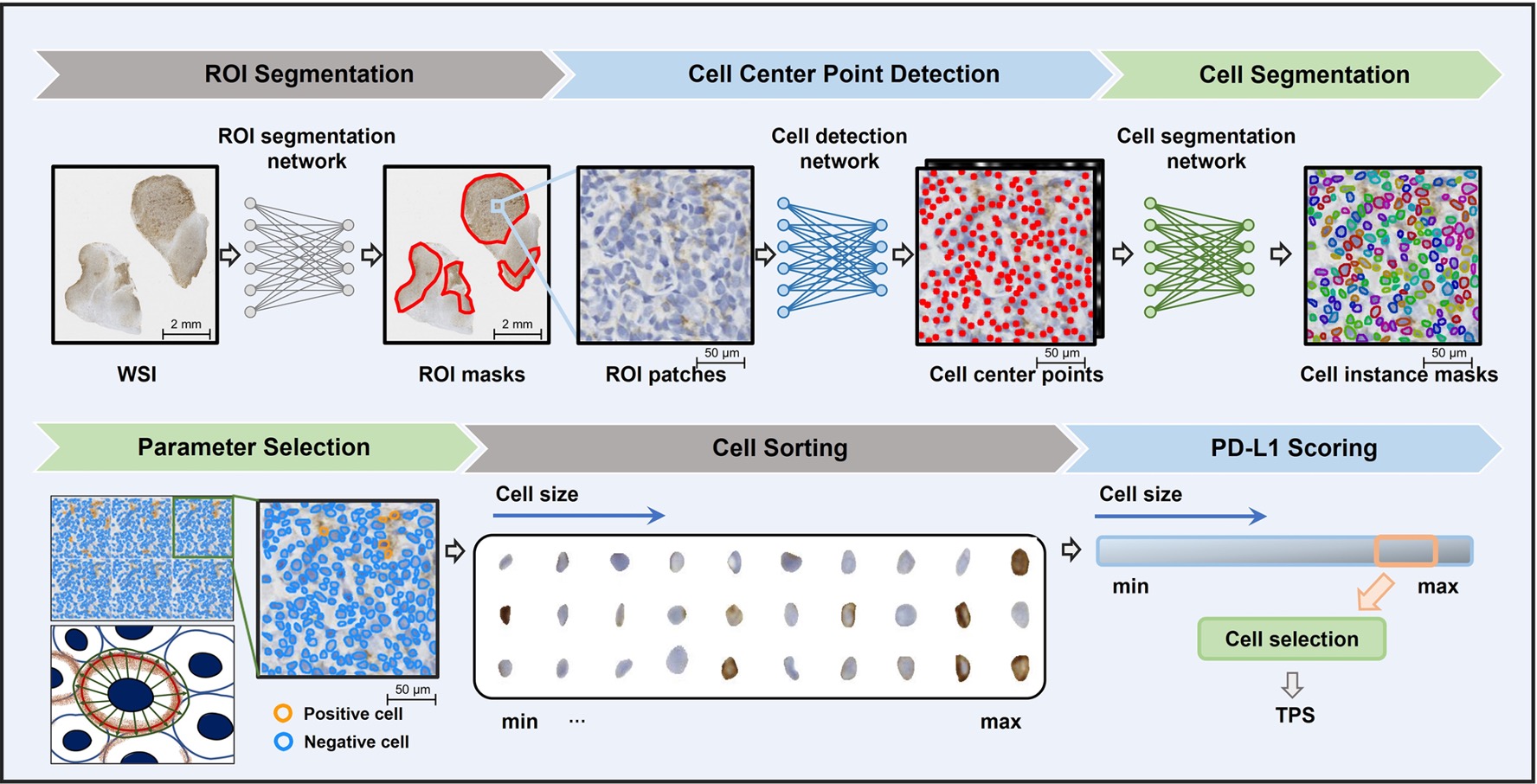
Foundation Model PathoDuet
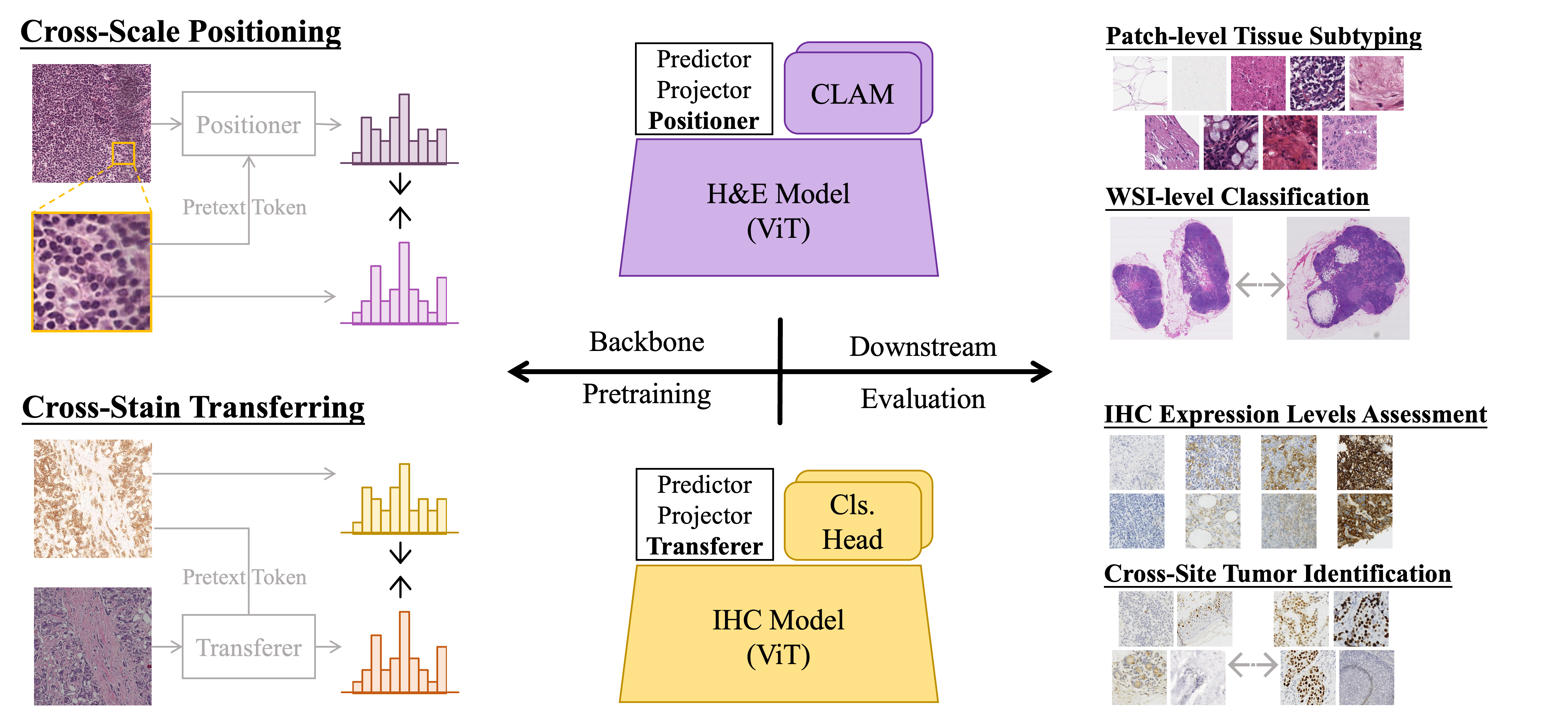
Here is the proposed self-supervised learning (SSL) framework. This framework aims at exploiting characteristics of histopathological images by introducing a pretext token during the training. The pretext token is only a small piece of image, but contains special knowledge. Code & Model
Foundation Model Adaptation PathoTune
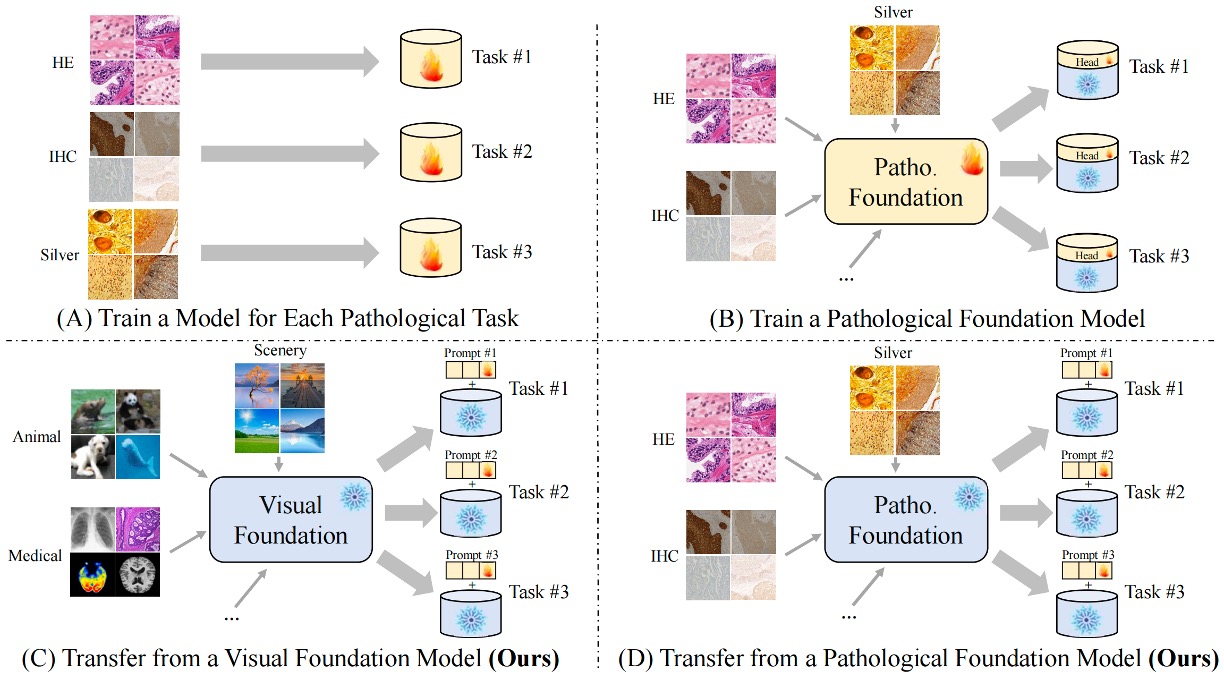
To mitigate foundation-task gap and the task-instance gap, we introduce PathoTune, a framework designed to efficiently adapt pathological or even visual foundation models to pathology-specific tasks via multi-modal prompt tuning.